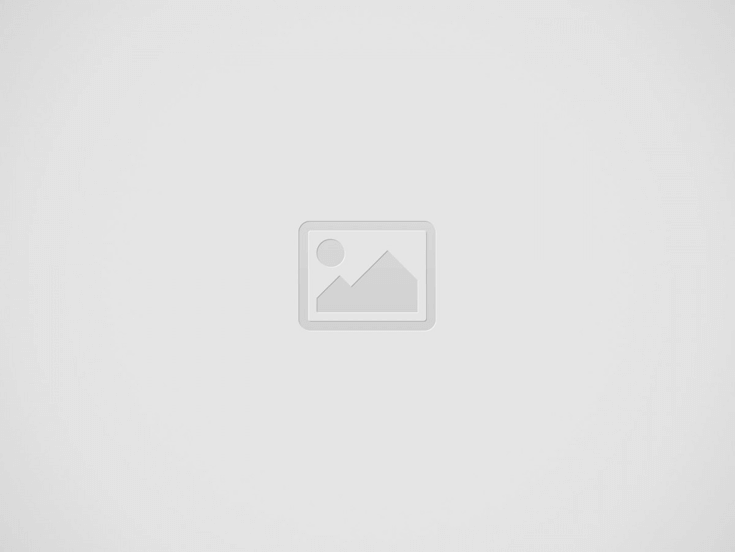
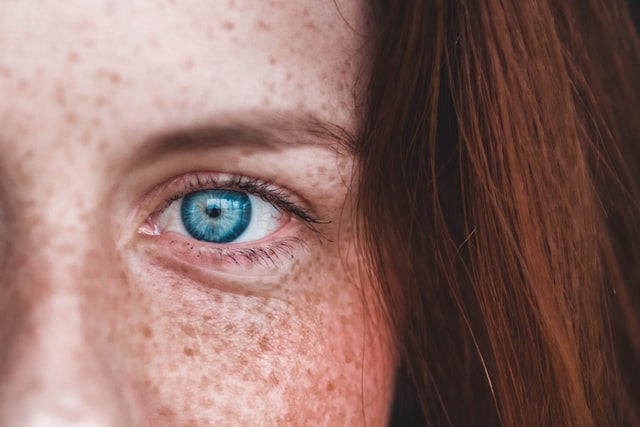
The AI bias trouble starts — but doesn’t end — with definition. “Bias” is an overloaded term which means remarkably different things in different contexts.
Here are just a few definitions of bias for your perusal.
- In statistics: Bias is the difference between the expected value of an estimator and its estimand. That’s awfully technical, so allow me to translate. Bias refers to results that are systematically off the mark. Think archery where your bow is sighted incorrectly. High bias doesn’t mean you’re shooting all over the place (that’s high variance), but may cause a perfect archer hit below the bullseye all the time. In this usage, the word carries little emotional connotation.
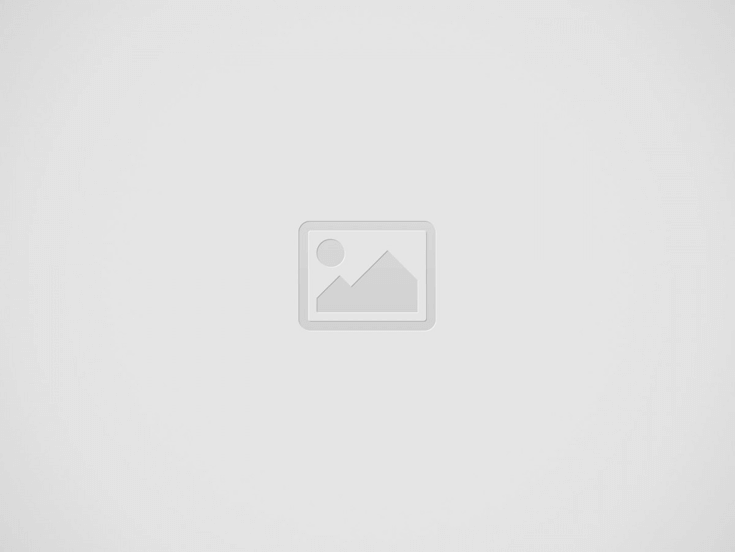
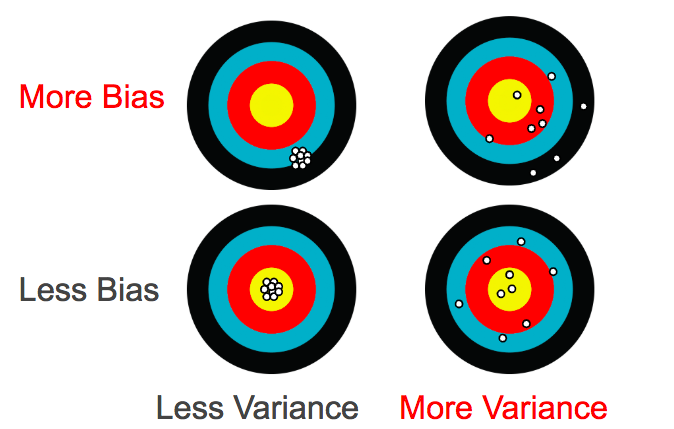
- In data collection (and also statistics): When you fumble your data collection so your sample isn’t representative of your population of interest. “Sampling bias” is the formal name here. This kind of bias means you can’t trust your statistical results. Follow this link for my article on it.
- In cognitive psychology: Systematic deviation from rationality. Every word in that pithy definition except “from” is loaded with field-specific nuance. Translation to layman’s terms? Surprise, your brain evolved some ways of reacting to stuff and psychologists initially found those reactions surprising. The list of cataloged cognitive biases is eye-popping.
- In neural network algorithms: Essentially, an intercept term. (Bias sounds cooler than that high-school-math word, right?)
- In the social and physical sciences: Any of a host of phenomena involving excessive influence of past/irrelevant conditions on present decisions. Examples include cultural bias and infrastructure bias.
- In electronics: A fixed DC voltage or current applied in a circuit with AC signals.
- In geography: A place in West Virginia. (I hear the French also have some Bias.)
- In mythology: Any one of these ancient Greeks.
- The one most AI experts think of: Algorithmic bias occurs when a computer system reflects the implicit values of the humans who created it. (Isn’t everything humans create a reflection of implicit values?)
- The one most people think of: The way our past experiences distort our perception of and reaction to information, especially in the context of treating other humans unfairly and other generalized badness. Some folks use the word synonymously with prejudice.
Oh dear. There are quite a few meanings here, and some of them are spicier than others.
Which one is ML/AI talking about?
The young discipline of ML/AI has a habit of borrowing jargon from every-which-where (sometimes seemingly without looking up the original meaning), so when people talk about bias in AI, they might be referring to any one of several definitions above. Imagine getting yourself prepared for the emotional catharsis of an ornate paper promising to fix bias in AI… only to discover (several pages in) that the bias they’re talking about is the statistical one.
The one that’s fashionable to talk about, though, is the one that gets media attention. The gory human one. Alas, we even bring all kinds of biases (past experiences that distort our perception of and reaction to information) along with us when we read (and write!) about these topics.
The whole point of AI is to let you explain your wishes to a computer using examples (data!) instead of instructions. Which examples? Hey, that’s your choice as the teacher. Datasets are like textbooks for your student to learn from. Guess what? Textbooks have human authors, and so do datasets.
Textbooks reflect the biases of their authors. Like textbooks, datasets have authors. They’re collected according to instructions made by people.
Imagine trying to teach a human student from a textbook written by a prejudiced author — would it surprise you if the student ends up reflecting some of the same skewed perceptions? Whose fault would that be?
The amazing thing about AI is just how un(human)biased it is. If it had personhood and opinions of its own, it might stand up to those who feed it examples dripping with prejudice. Instead, ML/AI algorithms are simply tools for continuing the patterns you show them. Show them bad patterns and that’s what they’ll echo. Bias in the sense of the last two bullet points doesn’t come from ML/AI algorithms, it comes from people.
Bias doesn’t come from AI algorithms, it comes from people.
Algorithms never think for themselves. In fact, they don’t think at all (they’re tools) so it’s up to us humans to do the thinking for them. If you’d like to find out what you can do about AI bias and go deeper down this rabbit hole, here’s the entrance.